

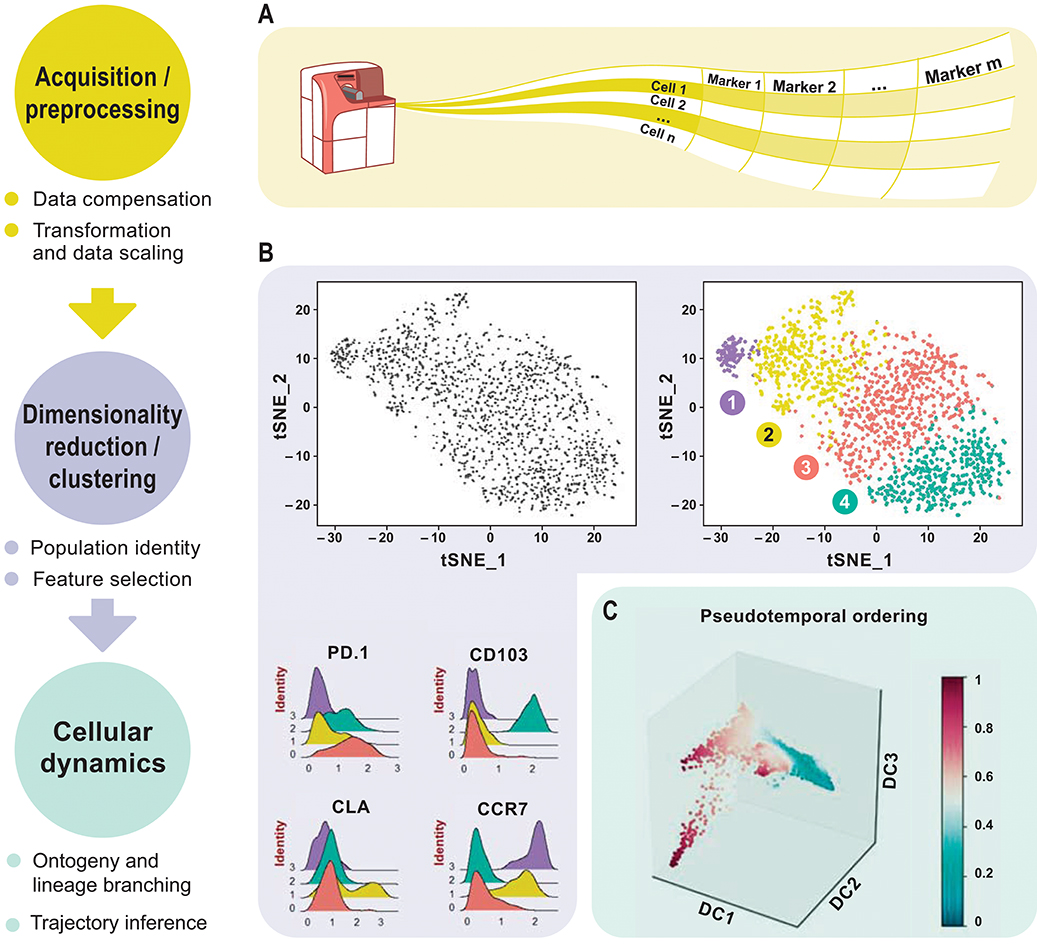
Workflow- Because tSNE relies on the initial state of events seeded into the algorithm, the output will not be identical for different samples.Therefore, you may see an improvement in your results if your data is on scale and whitespace is minimized. For more information on this subject, see our help pages on transformations in FlowJo v10. Data Scaling – The tSNE algorithm operates on data as transformed within your workspace.Inclusion of irrelevant parameters can add background noise in the calculation without contributing to the signal. Do not include parameters that may have been collected, but were not utilized in the staining panel and leave out any common parameters that do not vary across the sample population you are investigating. If your data set is fluorescence-based, select only compensated parameters (Comp-Parameter::Stain Reagent). Parameter Selection – In addition to choosing which events to use in your tSNE calculation it is also important to choose appropriate parameters.In addition, gate to include only the cells of interest (e.g., gating on CD3+ if T cells are of primary interest). This step reduces noise in the data and can improve the tSNE algorithm output. Cleaning up your data – The best analyses begin with cleaning up raw data to exclude doublets, debris, and dead cells.This section will briefly cover a few key points in the area of preparing your data.

While tSNE is a powerful visualization technique, running the algorithm is computationally expensive, and the output is sensitive to the input data. The native platforms in FlowJo (such as tSNE) do not require R.It can be accessed and run through the Populations menu (Workspace tab –> Populations band). įlowJo v10 has an extremely powerful native platform for running tSNE. Please see the references section for more details on the tSNE algorithm and its potential applications. It can be used independently to visualize an entire data file in an exploratory manner, as a preprocessing step in anticipation of clustering, or in other related workflows. Importantly, tSNE can be used as a piece of many different workflows. The tSNE-generated parameters are optimized in such a way that observations/data points which were close to one another in the raw high dimensional data are close in the reduced data space. T he tSNE platform computes two new derived parameters from a user defined selection of cytometric parameters. TSNE is an unsupervised nonlinear dimensionality reduction algorithm useful for visualizing high dimensional flow or mass cytometry data sets in a dimension-reduced data space. T-Distributed Stochastic Neighbor Embedding (tSNE) is an algorithm for performing dimensionality reduction, allowing visualization of complex multi-dimensional data in fewer dimensions while still maintaining the structure of the data.
